À propos d'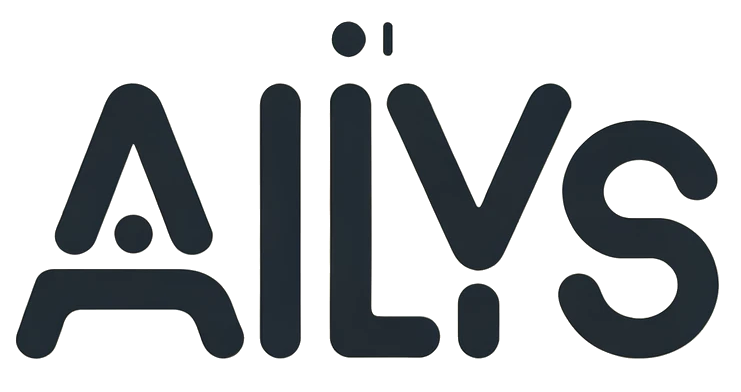
réunit les principaux acteurs académiques du site de Lyon et Saint-Étienne avec un consortium d'entreprises partenaires. Soutenu par la Région Auvergne-Rhône-Alpes, il vise à stimuler et approfondir la recherche, la formation et l'innovation au meilleur niveau en intelligence artificielle et pour ses usages.
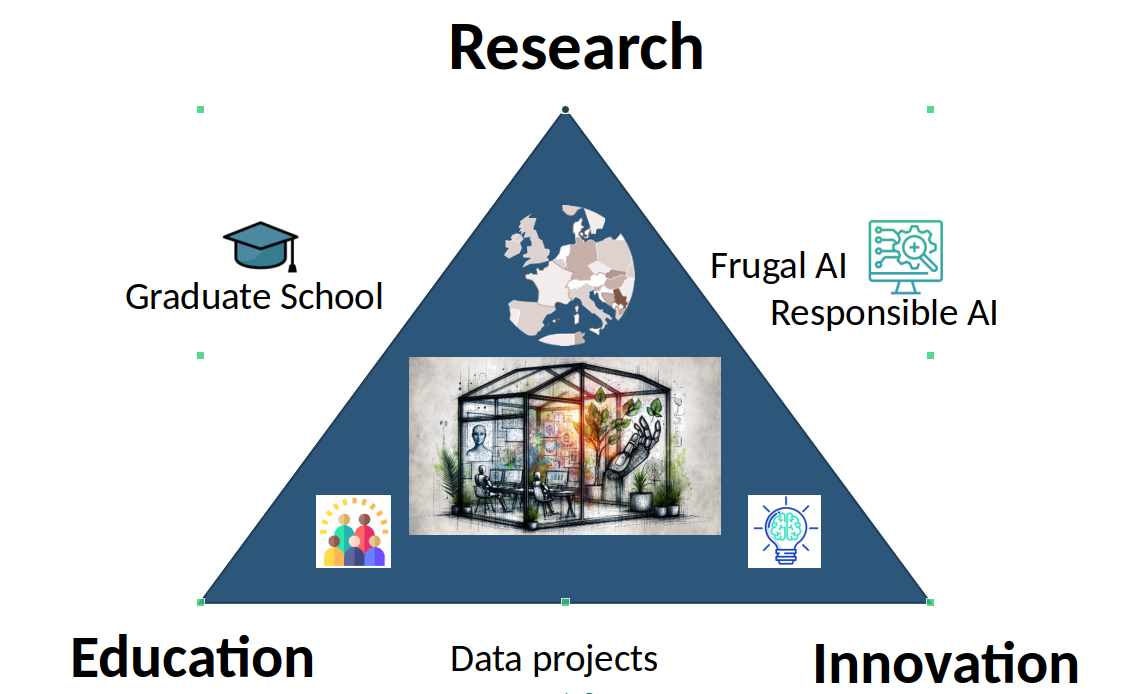
Programme 28 juin 2024
- 9h30 Accueil des participants
- 10h00 Towards Frugal Algorithms, Mathurin Massias
- 11h00 Homomorphic encryption for artificial intelligence, Jung Hee Cheon
- 12:00 Pause déjeuner (temps libre, il y a beaucoup de possibilités de restauration dans le quartier)
- 14:00 Inclusivité pour un TAL responsable, Aurélie Névéol
- 15:00 Table ronde: l'innovation IA en Auvergne-Rhône-Alpes, animée par Benoît Martin, avec Quentin Barthelemy Jérémy Espinas, Vincent Schanen, Damien Stehlé
- Temps 1 : Retours d’expérience
- Temps 2 : les challenges en cours, les besoins de chacun
- Temps 3 : Focus sur l'IA frugale : impacts environnementaux et sociétaux de l’IA
- 16:30 Session posters
- 17:30 Fin de la journée
Intervenants
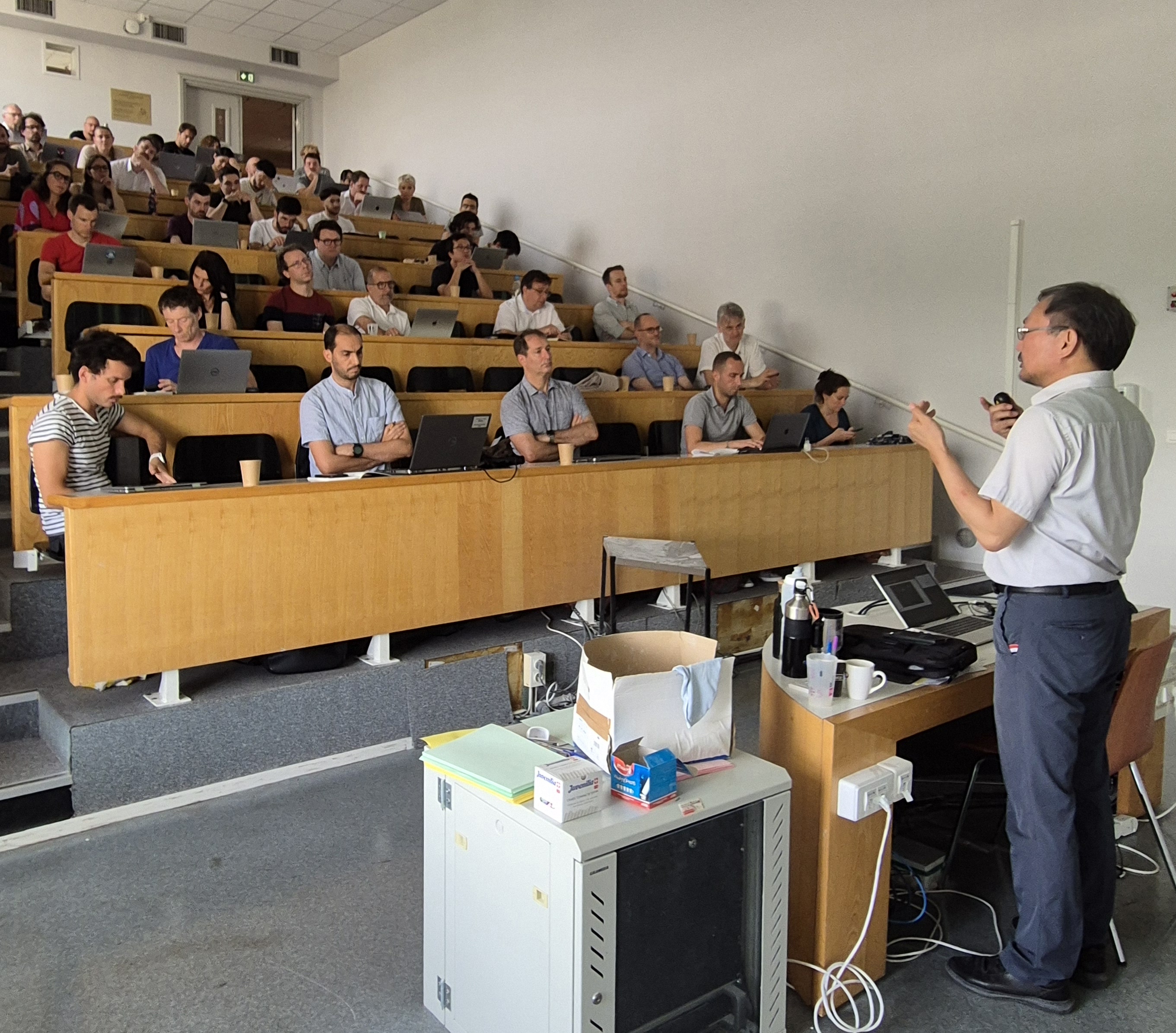
- Jung Hee Cheon, Department of Mathematics in Seoul National University, Director of Cryptographic Hard Problem Research Initiatives and creator of cryptolab
- Mathurin Massias, est chargé de recherche INRIA dans l'équipe Ockham, en poste à l'ENS de Lyon
- Aurélie Névéol est Directrice de Recherche au laboratoire LISN, et travaille sur le traitement des langages naturels pour les sciences cliniques et bio-médicales
- Benoît Martin est le responsable du PUI Impulse
- Quentin Barthelemy est responsable R&D chez Foxstream et spécialiste de frugalité
- Jérémy Espinas est ingénieur R&D chez Esker dans l’équipe en charge du développement de la majorité des fonctionnalités d’IA
- Vincent Schanen est responsable recherche et développement chez Syensqo
- Damien Stehlé est responsable scientifique chez Cryptolab France
Détails pratiques
Lieu: ENS de Lyon site Monod, amphithéâtre A (4ème étage)
Soumettre un poster: envoyer un lien vers le document pdf aux organisateurs
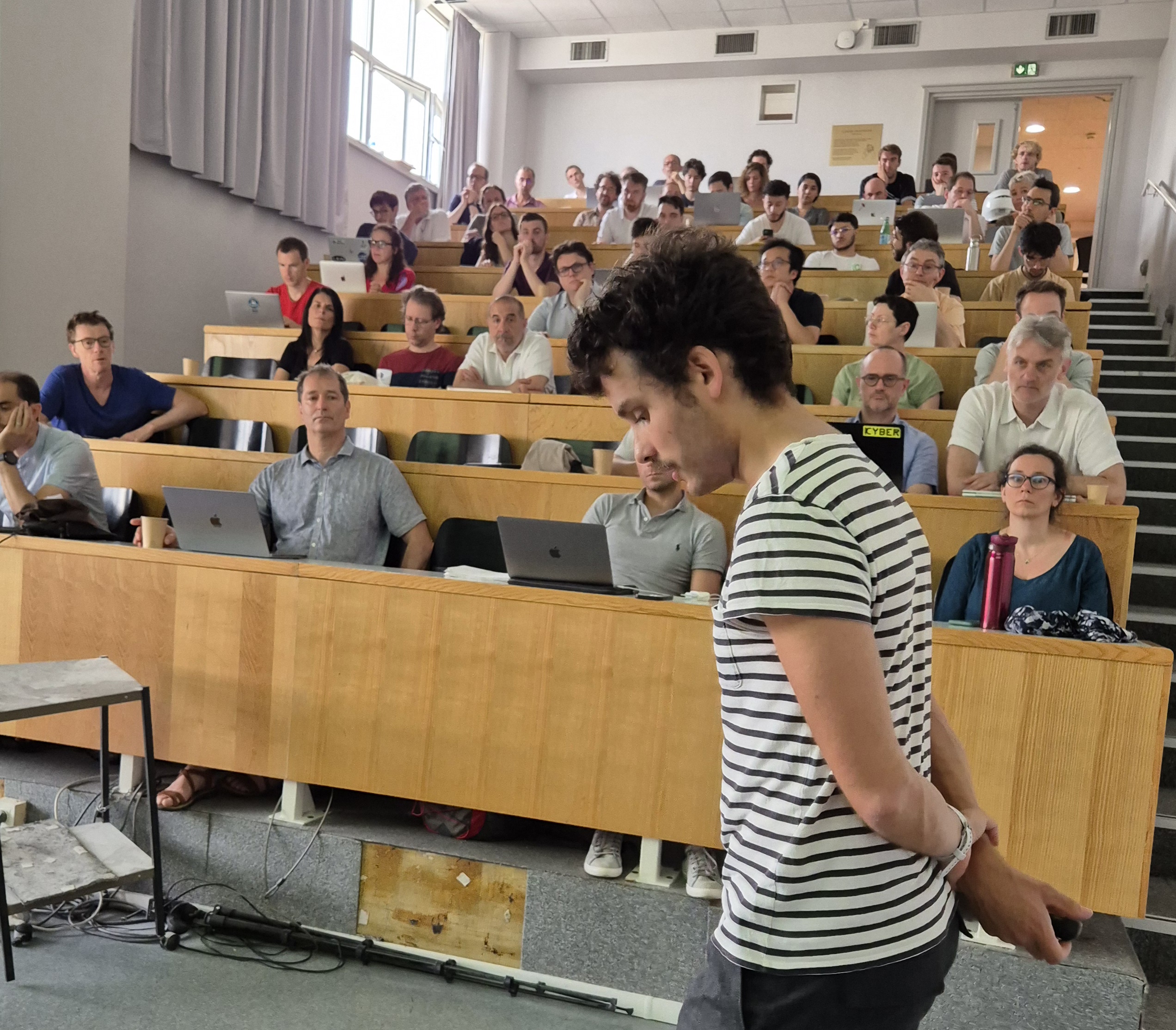
Inscription gratuite mais obligatoire
Les inscriptions sont closes.
Pour vous inscrire à la journée AILyS du 28 juin 2024, veuillez remplir le formulaire d'inscription ci-dessous.
Optimization for Artificial Intelligence - February 14th, 2025
Robustness, Overfitting, Transfer, Frugality.
Where ?
Ens Lyon 46 allée d'Italie, 69007 Lyon
- Amphi B de 8h30 à 12h30.
- Salle des thèses de 13h45 à 17h30
When ? February 14th, 2025.
When ? Aurélien Garivier (ENS Lyon), Rémi Gribonval (INRIA), Olivier Teytaud (Meta)
Program
Who ? | What ? | When ? |
Amphi B, 46 allée d'Italie, 69007 Lyon | ||
Introduction | 9:00 - 9:10 | |
O. Teytaud | Optimization for Post-Training in Large Language Models | 9:10 - 10:00 |
J. Tacchella | UNSURE: Unknown Noise level Stein's Unbiased Risk Estimator | 10:00 - 10:50 |
Coffee Break | ||
Cédric Gerbelot | Mean field theory for SGD “High-dimensional optimization for the multi-spike tensor PCA problem” | 11:10-12:00 |
Lunch break | ||
Salle des Theses, 46 allée d'Italie, 69007 Lyon | ||
R. Gribonval | Conservation laws during neural network training | 14:00-14:50 |
E. Ricietti | Frequency-aware training of PINNs : a multigrid idea | 14:50-15:40 |
G. Charpiat | Neural Network Growth | 15:40-16:30 |
The end ! | 16:30 |
Abstract de J. Tacchella: Recently, many self-supervised learning methods for image reconstruction have been proposed that can learn from noisy data alone, bypassing the need for ground-truth references. Most existing methods cluster around two classes: i) Stein's Unbiased Risk Estimate (SURE) and similar approaches that assume full knowledge of the distribution, and ii) Noise2Self and similar cross-validation methods that require very mild knowledge about the noise distribution. The first class of methods tends to be impractical, as the noise level is often unknown in real-world applications, and the second class is often suboptimal compared to supervised learning. In this talk, I will present a theoretical framework that characterizes this expressivity-robustness trade-off and propose a new approach based on SURE, but unlike the standard SURE, does not require knowledge about the noise level. I will also show that the proposed estimator outperforms other existing self-supervised methods on various imaging inverse problems.
Abstract de C. Gerbelot: A core difficulty in modern machine learning is to understand the convergence of gradient based methods in random, high-dimensional, non-convex landscapes. In this work, we study the behavior of gradient flow and online stochastic gradient descent applied to the multi-spike tensor PCA problem, the goal of which is to recover a set of spikes from noisy observations of the corresponding tensor. The main thrust of our proof relies on a sharp control of the random part of the dynamics, followed by the analysis of a finite dimensional dynamical system, leading to both sample complexity bounds and a complete description of the set of critical points reached by the dynamics. In particular, we obtain sufficient conditions for reaching the global minimizer of the problem from uninformative initializations. At a technical level, we will put our methods, originating in probability and mathematical physics, in perspective with those used in machine learning theory and statistical physics of learning. This talk is based on joint work with Vanessa Piccolo and Gérard Ben Arous.
Abstract de E. Riccietti: It is well known that the training of physics-informed neural networks (PINNs) may be difficult and slow in the presence of high-frequencies. Interestingly, the same phenomenon arises in the solution of partial differential equations by classical smoothing methods, just in the opposite direction: the low frequencies are difficult to reduce. Multigrid methods address this challenge by exploiting the complementarity between the involved sub-problems and achieve great acceleration and computational savings. In this work we propose an extension of the basic principle of multigrid methods to the training of PINNs to obtain a frequency-aware training scheme. We show that our approach is particularly effective if coupled with specialized frequency-aware network architectures.